About
Postdoctoral Research Associate / Computation and Informatics in Biology and Medicine (CIBM) Fellow at the University of Wisconsin Madison.
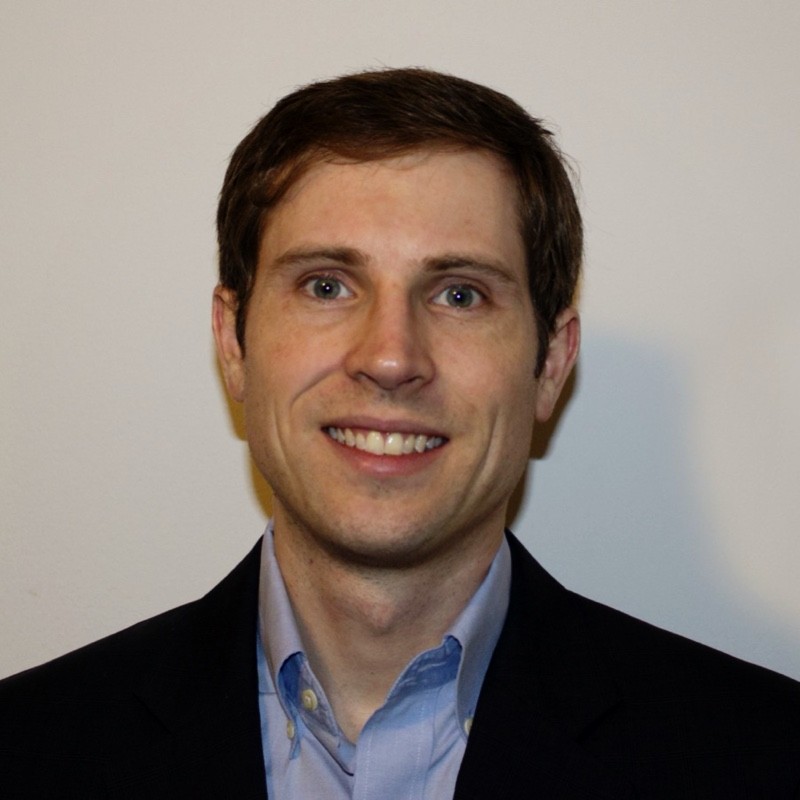
Drug Discovery, Bioinformatics, Computational Biology
Ten years of experience in both academic and industrial settings, working in highly multidisciplinary microbial natural product drug discovery (analytical chemistry, structure elucidation, biochemistry, microbiology). Experienced in both wet and dry lab techniques.
Experienced bioinformatician, designing and writing novel, complex software for Mass Spectrometry, Genomics, Metagenomics in R, Python, Nextflow, Bash, etc. Experienced in handlng large data, using high throughput computing, Unix, etc.
- LinkedIn: linkedin.com/hirechase
- GitHub: github.com/chasemc
- City: Chicago, USA
- Freelance: Available
Award Highlights
Download full CV.pdf for listing of all awards
-
Computation and Informatics in Biology and Medicine (CIBM) training (T32 institutional training fellowship)
- "The mission of the CIBM training program is to educate and mentor the next generation of leaders in biomedical informatics and biomedical data science research. The program is designed to equip and mentor predoctoral trainees to develop and apply novel, state-of-the-art computational methods for advancing biomedicine."
- cibm.wisc.edu/
-
NIH Predoctoral Individual National Research Service Award (F31)
- "The purpose of this Kirschstein-NRSA program is to enable promising predoctoral students with potential to develop into a productive, independent research scientists, to obtain mentored research training while conducting dissertation research."
- reporter.nih.gov/search/Kt67k3CPR0WtrcwRMl_upA/project-details/9759178
- W.E. van Doren Scholar
- American Society of Pharmacognosy Student Travel Award
- University of Illinois at Chicago Provost/Deiss Award
-
Bonner Scholar
- Four-year undergraduate community service scholarship requiring weekly and summer commitments
- About Bonner: www.bonner.org/goals
- berry.edu/admission/scholarships-and-aid/bonner
Total Funding and Scholarships
Skills
Under construction
Summary
Chase M Clark, Ph.D.
Education
Ph.D.; Pharmacognosy
2015 - 2020
University of Illinois at Chicago, Chicago, IL
Thesis: IDBac: A Proteomic and Metabolomic Platform for Microbial Drug Discovery (doi.org/10.25417/uic.14134700.v1)
- Natural product drug dicovery, specifically bacterial-derived antibiotics
- Conceived, learned to code, and built a bioinformatics program (R Shiny app) for rapid bacterial metabolomics analyses
- Isolated and cultured 1000's of bacterial isolates and performed natural product isolation and structure elucidation
- First or co-first author on four studies/manuscripts
- Mentored 11 undergradaute and graduate students leading to publishable studies
Bachelor of Science; Biochemistry
2008 - 2012
Berry College, Mount Berry, GA
Thesis: Electrochemical studies of enzymatically modified electrodes for the detection of glucose
Honors Program
Professional Experience
Postdoctoral Research Associate/Fellow
2020 - Present
University of Wisconsin-Madison, Madison, WI (Remote from Chicago)
- Funded through Computation and Informatics in Biology and Medicine (CIBM) training program (National Library of Medicine training grant)
- Developing genomic and metagenomic bioinformatics software
- Currently developing a "big-data" platform for analyzing 100s of thousands of genomes and millions of proteins
Predoctoral research and F31 Predoctoral Fellow
2015 - 2020
University of Illinois at Chicago, Chicago, IL
Research and Development Technician
2013 - 2015
Deerland Probiotics & Enzymes, Kennesaw, GA
- Assisted in the design and results analysis of three clinical studies
- Presented monthly projects to CEO, VP Sales and VP Science & Technology
- Head of method development and identification of raw materials to meet CFR 21, FDA requirements
- Developed identifications for over 140 materials, from enzymes and botanicals to minerals and additives.
- Overhauled the Method Development Department establishing inter-departmental responsibilities, improving and creating new SOPs, streamlining identification requests by establishing a formal request process and interactive database
- Strengthened and created new relationships with third-party vendors, labs, and universities.
- Controlled budget of method development for purchase of standards, consum- ables, equipment and third-party testing
- Mentored interns from Kennesaw Mountain High School magnet program
Portfolio
Under construction
Services
Under construction